Autonomous Learning Drones Successfully Fly Through Obstacle Course
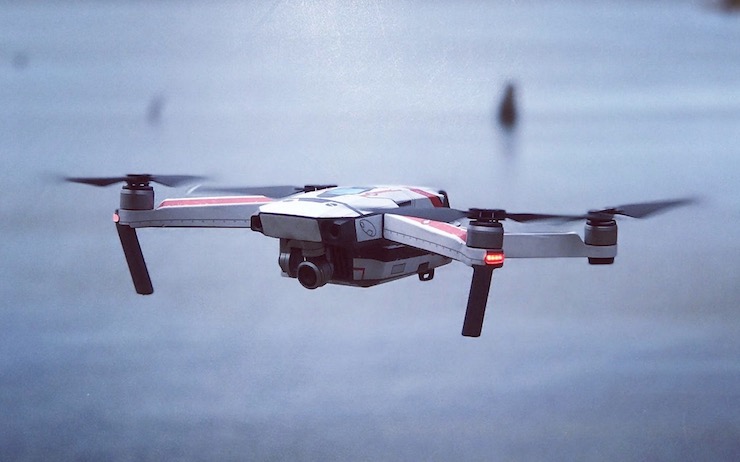
Drone racing has become one of the fastest growing entertainment sports. There is now a official organization, The Drone Racing League, that airs races on major networks like NBS Sports. Top ranking pilots aggressively race at speeds upwards of 90MPH through complex 3D courses. It is like taking an elite video racing game and setting it in a real world environment. It is a daring and adrenaline filled experience. But what if you were able to remove the human pilot from the scenario, using a drone that could completely navigate a course on it’s own? That is what one group of engineers from The University of Zurich are trying to do.
In 2012, under the leadership of Professor Davide Scaramuzza, the Robotics and Perception Group was founded as part of the Department of Informatics at the University of Zurich, and the Institute of Neuroinformatics. The goal of this group is to develop machines that can navigate through different environments with complete autonomy with just the use of a camera. Machines like cars have already proven that they can drive themselves because of the very advanced software they have on board. A self driving car uses a combination of GPS tracking and Lidar to navigate. These provide the car’s computer with a detailed static map and a way of measuring the current environment with pulsated lasers. A drone however is to small to be able to support such equipment.
That is why Professor Scaramuzza’s team is coming up with a way to train a drone’s neural processing to navigate through environments by visually learning them, much like how a drone pilot uses the drone’s onboard camera to see what’s ahead of it. They began the process by setting up several obstacle courses and slowly walking a drone through it so the camera could capture tens of thousands of images of it’s surroundings. Then they set the drone to fly autonomously through the course. Antonio Loquercio, one of Professor Scaramuzza’s PhD students who helped design the program explained, “The drone receives an image from the camera and the neural network outputs, Hey drone, now you have to go two meters to the left.”
As the drone moves through it’s course it is constantly relaying messages between it’s camera and neural network to make adjustments.
The program has been so successful that is was able to complete 50 out of 50 tests without a single crash. Because the drone isn’t using a static GPS system it can adapt to environmental changes. So even when the team added or moved obstacles in the drone’s path, it was able to adapt it’s trajectory and finish the course smoothly on it’s own. To further test their autonomous drone the team brought in a top ranked pilot from the Drone Racing League to compete against.
The drone by the Robotics and Perception Group again maneuvered through 50 out of 50 laps of the course while the professional pilot completed 45 out of the 50 laps without incident. Loquercio was quick to point out one major difference between the drone flying autonomously versus the one piloted, speed. “Drone pilots fly very, very aggressively,” he said, “they are more open to take risks, way less conservative than what our current approach is.” In fact the drone pilot flew at least three times faster than the one developed by Professor Scaramuzza’s students.
As of right now, The Drone Racing League has nothing to worry about. Because they are limited to slower speeds to complete the neurological paths needed for success, it doesn’t seem that any autonomous drones will be winning any of their races anytime soon. However, this breakthrough has plenty of other applications. The drones systems designed by the Robotics and Perception Group are so accurate that they could likely solve the logistical issues of drone deliveries in populated areas. Because they rely on neural learning rather than just a GPS map they could easily navigate through cities and towns safely.
|